When it comes to collecting data, you want to make sure that your survey responses are statistically significant. A few factors can go into this, including sample sizes and how many respondents are needed – all of which are tested for validity to ensure statistical significance.
But how do you determine what an appropriate sample size is? How many survey responses should you be aiming for in order to achieve statistical significance?
We’re looking at some general guidelines for understanding statistical significance and determining the ideal number of survey responses needed for your research project.
We’ll also touch on factors such as confidence levels, the margin of error, population size, and more factors that go into determining statistical significance!
Important Statistical Significance Terms
Before diving into the ins and outs of statistical significance, it’s important to know the relevant terms. Below is a brief overview of just a few.
Confidence Level
The confidence level is the percentage of accuracy that you are looking to achieve with your survey responses. Typically, a confidence level of 95% or higher is desired in order to ensure statistical significance. This means that you have a 95% chance that your results will remain accurate and reliable if the same survey were administered again.
Margin of Error
The margin of error is the range in which you can expect your survey results to fall with a given confidence level. For example, if the margin of error for a survey is +/- 5%, then you could expect the true value to be within that 5% range.
Population Size
Population size is important to consider when calculating the necessary number of survey responses. The larger the population, the larger your sample size needs to be in order to achieve statistical significance.
P-Value
The p-value is the probability that a given result would occur by chance. If the p-value is low (e.g., 0.05 or lower), then it indicates statistical significance as there is only a 5% chance of such results occurring randomly.
Sample Size
Sample size is the total number of responses that are collected in a survey or experiment. It is one of the most critical factors to consider when assessing statistical significance, as it determines the accuracy of a given set of results. Ideally, sample size should be large enough to provide an accurate representation of the population being studied, while still being small enough to be manageable.
Sampling Error
Sampling error is the difference between the results of a sample and the true (but unknown) value in the population. It can be caused by factors such as selection bias, non-response bias, and more.
What is Statistical Significance and Why Is It Important?
Statistical significance is the likelihood that results from your survey are not due to chance. For instance, if you’re surveying a random sample of people and find that 90% approve of a certain policy, you can be more confident that the result is statistically significant and not just an anomaly.
In other words, statistical significance helps researchers make decisions with more certainty.
To calculate statistical significance, researchers use a combination of factors such as confidence levels, margin of error, and population size.
The Difference Between Statistical Significance and Practical Significance
Because these terms are often used interchangeably, it’s important to remember that statistical significance is not the same as practical significance.
Statistical significance means that your results are likely true and accurate, but they may not have any meaningful implications in the real world.
Practical significance refers to data that has practical implications for decision-making or policy-setting, which is largely different than the intent of statistical significance.
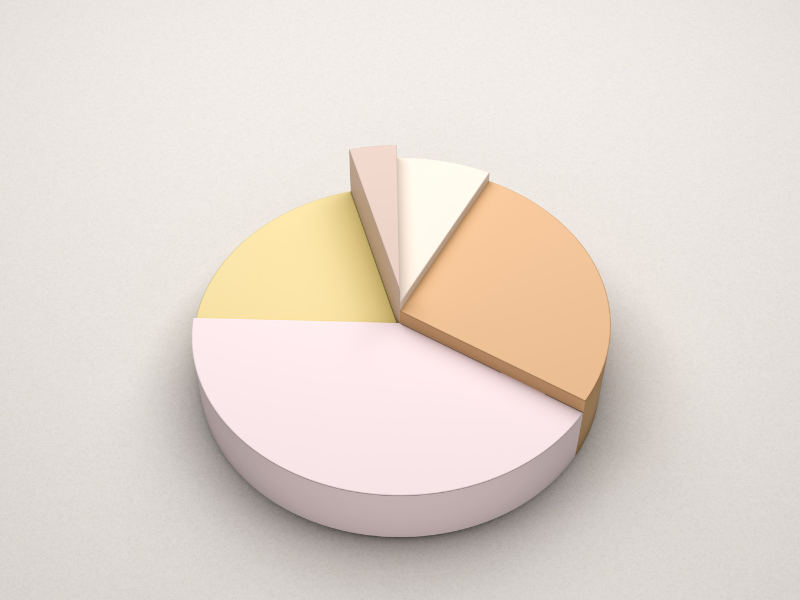
How to Calculate Statistical Significance
Determining statistical significance is a multi-stepped process. Usually, the process will begin with creating a null hypothesis and evolve from there.
Create a Null Hypothesis
To calculate statistical significance using the null hypothesis, researchers first must define the statement they are trying to prove (the null hypothesis). The null hypothesis states that there is no difference between two sets of data and that any observed differences are due to chance alone.
Determine an Alternative Hypothesis
While your null hypothesis is generally negative and the opposite of your intent with your data, the alternative hypothesis is the reason for your research. The alternative hypothesis says that your data is significant.
Analyzing the Statistical Significance Level
The next step is to gauge whether the significance level is above or below the threshold of .05 – 5%, depending on the research field.
The next steps include calculation via standard deviation and standard error formulas to understand whether collected data is in fact statistically significant.
For more information on null hypothesis testing and its relationship with statistical significance, read Null Hypothesis Significance Testing: A Short Tutorial
So now that we know how important sample sizes are for ensuring statistical significance, how can you ensure your study meets the threshold?
We’re breaking down a few tips for establishing a solid sample size to achieve statistical significance.
How to Calculate the Sample Size Needed for Your Survey
Calculating the ideal sample size for a survey is not always an exact science. Some recommend 100-200 responses at a minimum to ensure statistical significance but that is not always logical for smaller businesses or mega-corporations.
However, bigger surveys are even better; more responses mean greater precision and accuracy in your data.
When determining the sample size for your survey, you should also take into account factors such as the population size, confidence level, and margin of error.
For example, if you’re surveying a large population with a 95% confidence level and a 5% margin of error, you’ll need to collect more responses than if the population were smaller and the confidence level were lower.
It’s also important to consider the impact of non-response bias and other factors that can affect the accuracy of survey results.
Non-response bias occurs when some groups are underrepresented in your sample, which can lead to inaccurate results if not accounted for.
To help prevent this, researchers recommend sending out survey requests based on the assumption that only 20% of respondents will answer. So in the case that you need 20 responses, assume you will need to send out 100 or more requests.
Factors That Impact Sample Size Requirements
The sample size requirements for a research study are determined by the factors such as:
- Research type: Depending on the design of the research, it can have an impact on how large or small a sample size needs to be in order to get meaningful results. For example, if a study is looking at certain correlations between variables, a larger sample size may be necessary to detect those relationships.
- Population size: As mentioned above, the population size can influence the size of the sample needed for statistical significance. The larger the population, the more responses you’ll need to accurately represent it.
- Confidence level: A higher confidence level requires a larger sample size in order to reach statistical significance.
- Margin of error: The margin of error affects the sample size as well since a lower margin of error requires more responses for accuracy.
By taking all these factors into consideration, researchers can determine the ideal sample size for their surveys and experiments and obtain reliable results that can be used to inform decisions.
Benefits of Increasing Your Response Rate
Increasing your response rate can have a number of benefits for researchers. First and foremost, it allows you to obtain more accurate data with greater precision and confidence.
A higher response rate also reduces the risk of non-response bias, which can lead to inaccurate results if not taken into account.
Additionally, the larger sample size can provide a better representation of the population, allowing for more accurate generalizations.
Overall, a higher response rate can help researchers make better decisions with greater confidence in their results.
By taking into account all the factors that impact sample size requirements, researchers can determine the ideal number of responses needed to get reliable data from their surveys and experiments.
Additional reading: Qualitative vs Quantitative Research: Which Is the Best Research Method?
Tips for Improving Your Response Rate and Gathering More Data
While there is no single full-proof way to increase your response rate, you can certainly trial-and-error some different approaches to see which people respond to best.
Because every business and its relationship with respondents is different, you’ll want to find what works best for you.
1. Personalize your emails and communication to show that you care about the respondent.
2. Offer incentives such as gift cards or discounts in exchange for survey responses.
3. Make sure your surveys are well-designed, organized, and easy to understand so respondents don’t get overwhelmed by long questions or confusing language.
4. Provide clear instructions on how long the survey should take and why their feedback is important to you.
5. Reach out multiple times using different channels (e-mail, SMS, social media) if needed to increase response rates from participants who have not responded yet.
6. Use follow up messages with reminders of deadlines or new information relevant to the research topic/questionnaire which could motivate people to respond faster
7. Use a variety of question types that allow for open-ended answers along with more structured questions like Likert Scales & Multiple Choice Questions
8. Keep an eye on timing – sending surveys too late can decrease response rate significantly!
9. Track email metrics (open rate, click-through rate etc.) regularly and adjust where necessary
10. Encourage referrals from existing respondents who might know someone else interested in taking part in your survey
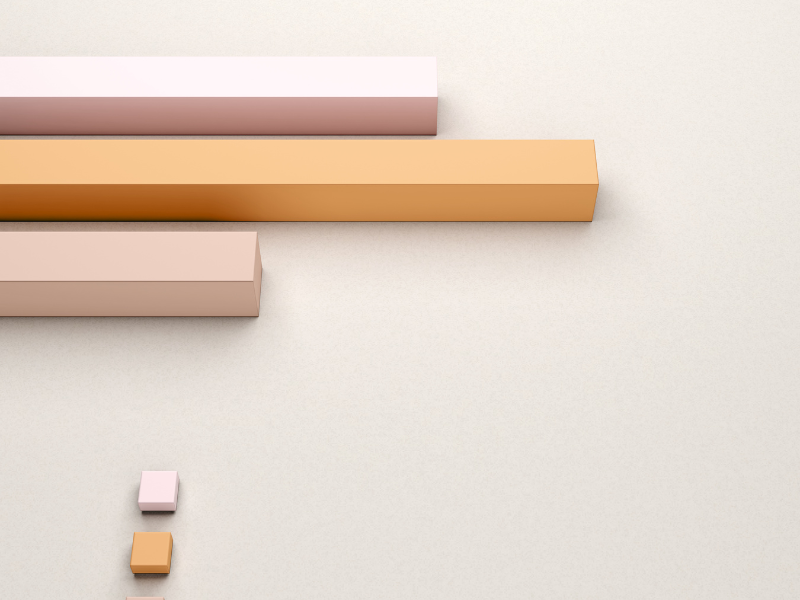
What to Do if Your Data Is Non-significant
The dreaded reality for many researchers: the research they’ve spent days, months, maybe years collecting is not statistically significant. Now what? There are generally two options.
A study’s research results showing that there is no statistical significance does not necessarily void the study’s validity or make it a “failure.”
Sure, when a null hypothesis is proven, it can signify that the central hypothesis of the study is incorrect, but it can also just signify a problem with the study, such as there not being enough data for a conclusive result.
One study explains,
“We should not conclude that the null hypothesis is true, but rather we must retain it as a possibility as we do not have enough evidence to reject it… We should consider all these possibilities along with the likelihood that there is no effect. The researcher has little control over the effect size and sample variation, although it is essential that these are reported for non-significant results.”
Reporting non-significant results also provides value to future researchers who intend to run similar surveys so they don’t make the same mistakes or flat-out repeat the study.
“…it is important to report all findings, significant or not, of well-designed studies for both effective dissemination and to minimize publication bias. Under-reporting of negative results leads to bias of meta-analyses, wastes resources as others replicate previous studies. There is also an ethical obligation to report studies using human subjects who have volunteered themselves usually at some risk to benefit others.”
The earnestness of being important: Reporting non-significant statistical results
Final Thoughts on Understanding Statistical Significance in Surveys
Understanding the concept of statistical significance can help researchers ensure that their survey results are as accurate as possible and free from bias.
By taking into account factors like population size, confidence level, and margin of error, researchers can determine the ideal sample size for their surveys to increase response rates and obtain reliable results.
Finally, by implementing some simple tips such as personalizing emails, offering incentives, and providing clear instructions, researchers can further help increase their response rate and gain more accurate data for their research.
In sum, by properly understanding the concept of statistical significance, researchers can build a strong foundation for gathering reliable survey results that are free from bias.
This will not only help them make better decisions in their research, but it could also make them more competitive in the field.